Types of Generative AI
A subset of artificial intelligence called generative AI involves producing new things depending on constraints or inputs. Numerous applications have been used, including creating voice and pictures, music, and even video games. We will give a thorough explanation of the various generative AI types in this blog article.
- Variational Autoencoder (VAE)
Generative AI of the Variational Autoencoder (VAE) type is frequently employed to create images. VAEs first learn a latent representation of an existing image to create new pictures. By adjusting the values of the latent variables, it is possible to create unique pictures from the compressed image form known as the latent representation.
- Generative Adversarial Networks (GANs)
A form of generative AI known as generative adversarial networks (GANs) combines a discriminator and a generator neural network. The discriminator separates between genuine and false material, while the generator produces new content.
The generator is instructed to produce fresh material that the discriminator cannot identify from genuine content while being fed random noise during training. A constant cycle of improvement results as the discriminator and generator get better.
- Recurrent Neural Networks (RNNs)
Generative AI techniques called recurrent neural networks (RNNs) are frequently employed for text production. RNNs produce new text by identifying patterns in a given dataset through learning. RNNs are particularly helpful for creating content with a predetermined structure, like poetry or song lyrics.
- Transformer Networks
Transformer Networks is a generative AI class frequently employed for text creation and translation tasks in natural language processing (NLP). Transformer Networks function by selecting the input portions to concentrate on when producing output utilizing a self-attention process.
Transformer Networks are particularly effective in producing long-form material, like research papers and news pieces.
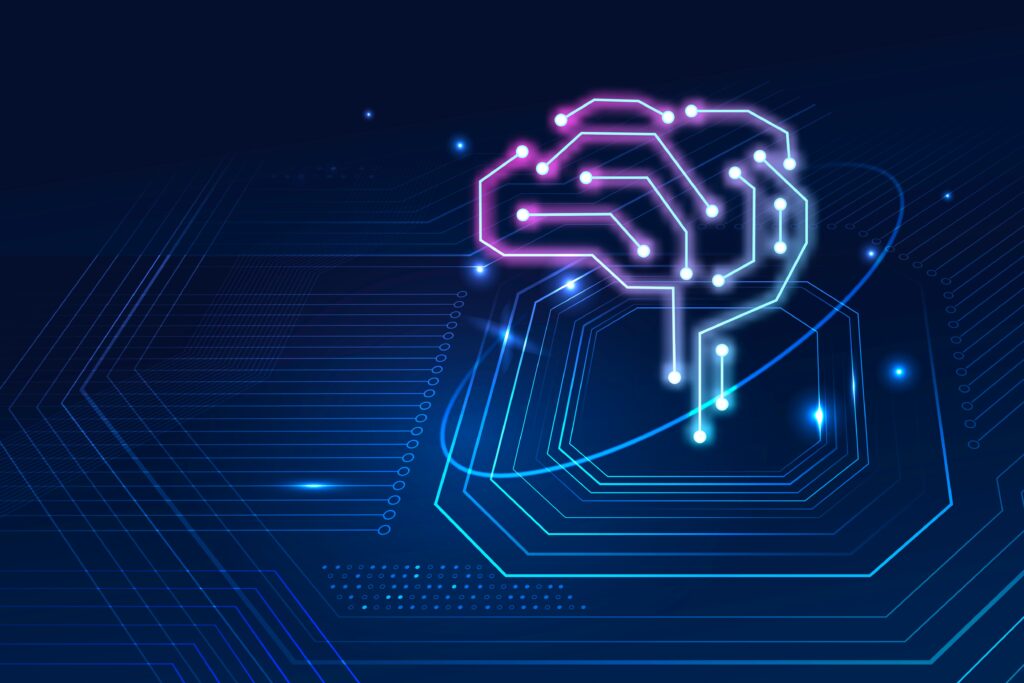
- Deep Belief Networks (DBNs)
An example of generative AI is Deep Belief Networks (DBNs), frequently employed for unsupervised learning tasks like anomaly detection and recommendation systems. A particular dataset’s underlying patterns are discovered via DBNs, which may be utilized to create new data. DBNs are very helpful for creating fresh content in industries like music and art.
- Autoencoders
An example of generative AI is an autoencoder, frequently employed for picture creation and compression. Autoencoders create new pictures by first learning a compressed version of an existing image. Autoencoders are very helpful for creating brand-new pictures that resemble current ones.
- Boltzmann Machines
An example of generative AI is the Boltzmann Machine, frequently employed for unsupervised learning tasks like picture production and categorization. To create new data, Boltzmann Machines simulate the interactions between the neurons in a given dataset. In fields like music and art, Boltzmann Machines are extremely helpful for creating new material.
- Convolutional neural networks
An example of generative AI is convolutional neural networks (CNNs), frequently employed for picture production and categorization. Convolutional layers are utilized by CNNs to learn the features of a given picture, which may subsequently be used to create new images.
CNNs are very helpful for image classification jobs and for creating new pictures comparable to current photos.