What is Generative AI?
In the vast world of artificial intelligence (AI), there are various subfields and approaches that aim to mimic human-like intelligence. One such fascinating area is generative AI, which focuses on creating AI models capable of generating new content, such as images, text, music, and even videos. Generative AI has gained significant attention and has showcased remarkable advancements in recent years, allowing machines to produce creative and original content.
Generative AI refers to a class of algorithms and models designed to generate new data that resembles a given training dataset. It involves training models to learn the underlying patterns, structures, and features of a particular dataset and then using that knowledge to generate new content. These models are typically trained on large datasets and can capture complex relationships and distributions within the data.
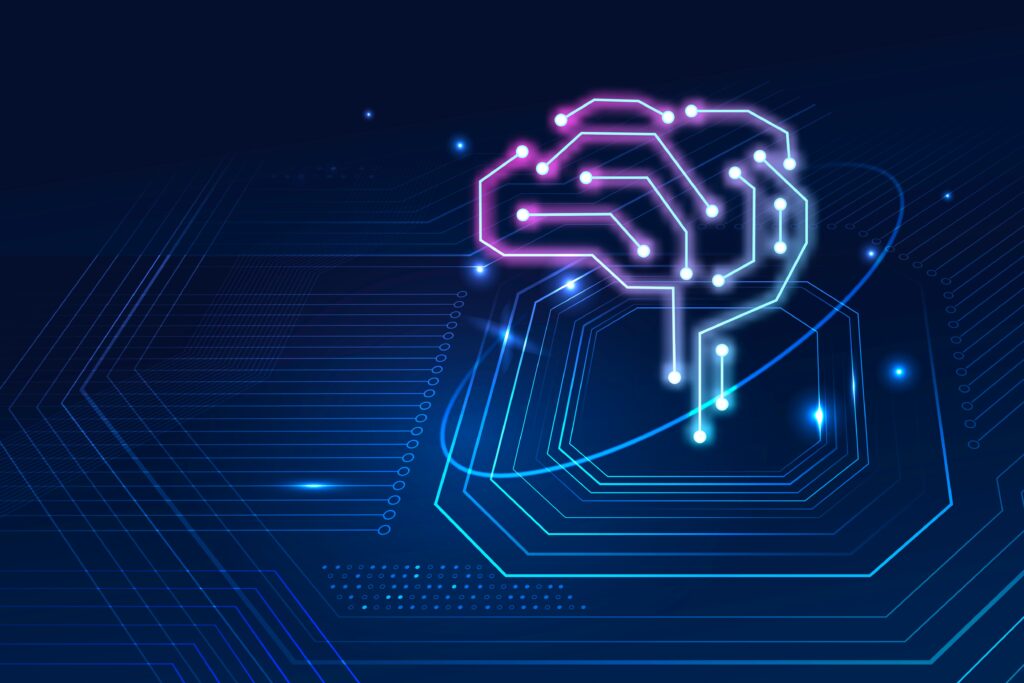
One of the fundamental techniques used in generative AI is generative adversarial networks (GANs). GANs consist of two main components: a generator and a discriminator. The generator tries to generate realistic data, while the discriminator’s role is to distinguish between the generated data and real data. Through an iterative process, the generator learns to improve its output by fooling the discriminator, and the discriminator becomes better at distinguishing real from generated data. This back-and-forth competition leads to the generation of increasingly realistic content.
One of the most popular applications of generative AI is in the field of computer vision. Generative models can generate highly realistic images that resemble the training dataset. For example, a generative model trained on a dataset of celebrity faces can create new images of faces that look convincingly real, even though they have never existed before. This technology has immense potential in fields like entertainment, gaming, and even law enforcement, where it can aid in creating realistic avatars, generating visual content, or assisting in facial recognition tasks.
Another area where generative AI has shown remarkable progress is natural language processing (NLP). Language models such as OpenAI’s GPT (Generative Pre-trained Transformer) have revolutionized the field by generating coherent and contextually relevant text. These models are trained on vast amounts of text data and can generate human-like responses, write articles, and even compose poetry. Generative AI in NLP has applications ranging from chatbots and virtual assistants to content creation and language translation.
Music generation is yet another exciting application of generative AI. AI models can analyze vast collections of musical data and learn to compose new melodies, harmonies, and rhythms. These generative music models have the potential to aid musicians and composers in the creative process by providing fresh ideas or assisting in generating royalty-free background music for various media projects.
While generative AI has brought about remarkable advancements and possibilities, it also raises ethical and societal considerations. For instance, the ability to generate highly realistic images and videos raises concerns about misinformation and the potential for misuse. Deepfakes, which are manipulated videos that replace the original person with someone else’s face, can have serious implications for identity theft, reputation damage, and the spread of false information.
To address these challenges, researchers and developers in the field of generative AI are actively working on techniques for detecting and mitigating deepfakes. They are also exploring ways to make the generated content more transparent, allowing viewers to differentiate between what is real and what is artificially generated.
In conclusion, generative AI is an exciting and rapidly advancing field within the realm of artificial intelligence. Through techniques like GANs, it enables machines to generate new and creative content that resembles the training data. From computer vision to natural language processing and music generation, generative AI has showcased its potential in various domains. However, it also poses ethical challenges, particularly in relation to the creation of deepfakes. As the field continues to progress